Many brands under categories like consumer appliances, cold beverages and ice-creams, healthcare, travel, auto associate themselves to IPL and gain upto 30-40% of their annual sales target in this period. Reach and width of IPL bolsters the brand perception and gives confidence to the audience and dealers about the product and thus sales increases. Seasonality is another factor that plays a major role in driving the sales.
But what constitutes ‘evidence’ that IPL marketing causes higher sales? How many sales are coming from IPL ? What would be the sales if there were no IPL ?
These are tricky questions with no one right answer. Most of the time, marketing analysts, research agencies and 3rd party teams are trying to work out the impact of marketing. It’s an important question, as the answer to this would help to plan the next investment in IPL.
If there exists a single media metric that would help us understand the effectiveness and efficiency of every media then the world would have been different. But in reality there is no one media metric which can be used to measure all media channels. Each media channel has a different role throughout the marketing funnel and needs to be evaluated on an appropriate metric basis its objective.
But the key question remains constant. How do we evaluate our campaign ? How do we know what’s working and what’s not ? How can we spend our next dollar more efficiently ?
So let’s try and understand the solution hierarchy that can help us answer aboves questions. In these solutions, some are better than others, but aren’t always applicable. So we have to look at the scenario and then point to the right solution accordingly.
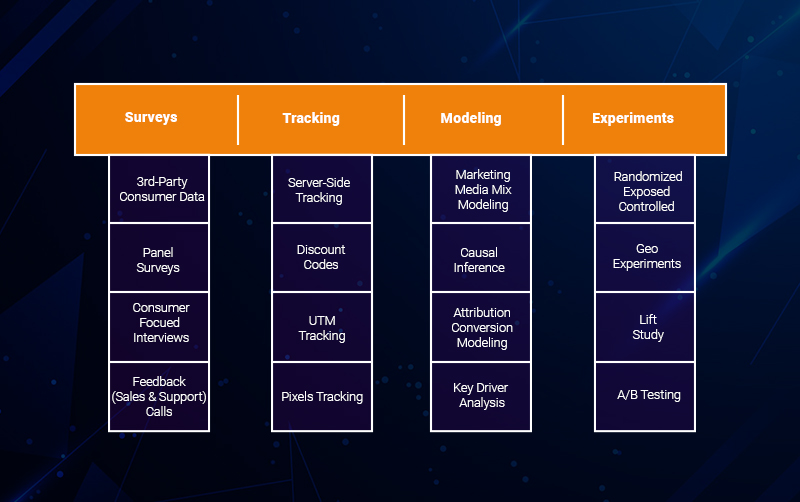
Tracking
Here, you want to know which digital channels are converting more, which is basically an attribution problem. This is known as the bottom up approach as you start from the most granular data and then aggregate it for business use-case. It is easy to set-up or understand.
Some of the challenges with this approach are
- Over and under attribution: Generally, touch based approaches, over-credits the “bottom of the funnel” channels i.e. FB, Google, Re-marketing etc and under-credits top of the funnel channels i.e. TV, Radio etc.
- Unique Reach problem: In digital marketing, if an audience is exposed to multiple channels, then each channel will take its credit. So one needs extra tracking layers i.e. UTM tags etc or support from 3rd party tools i.e. data.ai etc.
- Attribution models suffer from the problem that correlation does not imply causation and marketers are more interested in the causation. What will happen if they decide to increase their budget.
Experiments
Experiments create randomly assigned treatment and control groups, to estimate causal effects, a gold standard methodology to measure true incrementality. Generally there are two kinds of experiments i.e. “intra-channel” and “inter-channel” tests.
“Intra-channel” tests aim to identify the best version of an ad, within that same channel. It tells you, for example, which creative can work well, but it doesn’t tell what would happen if we increase the budget on creative by 10%
“Inter-channel” tests aim to measure the effect of changing the levels of spend for channels relative to each other. It helps in planning and budgeting.
Let’s also understand the challenges of this methodology
- Theory Vs Practicality gap: Setting up these methods require substantial pre-planning and resources and most of the time, measurement is an afterthought. Also business needs precedes measurement. So setting this experiment with control and exposed is difficult at times.
- Capability: Requires substantial man power with right talent to run. In practice, this can be a non-trivial amount of work, and many teams don’t have the capacity or expertise to make it happen very frequently.
- Missing / Lack data: It requires historical data for a decent period.If anything is missing then it is not feasible to create these experiments.
Surveys
Companies spend millions of rupees to increase their awareness by 1 or more percentage points. How do they measure these awareness numbers? Mostly they are being done by 3rd party agencies i.e. Kantar , Nielsen etc. They are already experts in this field. Brands can go either a complete manual, online panel or combination of both.
Idea is to hear directly from the horse’s mouth, about the success of a campaign. You reach out to your audience, ask them questions and then again repeat it with fixed frequency to understand if any metric is showing the movement or not.
At the start, top of the funnel metrics i.e. top of the mind awareness, consideration and intent is in focus. As a company reaches maturity, focus shifts to the bottom of the funnel business metrics i.e. installs, transactors etc.
Competition benchmarking is also done using these surveys
Modeling
This brings us to the final pillar: regression-based approaches. Like attribution models, they work with observational data, so they require less disruption to your existing business. And like experimental methods, their goal is to estimate causal effects, not just correlations.
Regression approaches take as their input time series of channel-level spend, control variables (like seasonal indicators), and of the relevant conversion metric (say, new customers acquired), and output estimates of the incremental effect of additional dollars spent.
Not only that, but different variations of these models estimate lagged effects, cross channel effects, geographic-level effects, and even more as well!
If this sounds too good to be true, the catch is that these models are enormously complex, require lots of assumptions, have a huge number of parameters to fit, and must do so with limited data. This makes them tricky to estimate, unstable (they give different results when the data is changed only slightly), and difficult to validate.
What’s the right mix?
Aiming for precision rather than accuracy.
Many companies are already employing a mix of all three approaches. How should they be integrated in a principled way? While there’s no right answer, we think about it in the following way:
First, we should remember that all this measurement is in service of figuring out how to allocate our marketing budget this week, this month, or this quarter. While, in a vacuum we may wish to get the best numbers possible, in the nitty gritty of the real world, the numbers need to be both actionable and timely.
This unfortunately precludes experimentation from forming the foundation of a marketing effectiveness system, since it is often too slow to fit inside the budgeting cycle.
Second, all three of the remaining approaches (surveys, attribution and regression) have weaknesses: attribution in that it is modeling correlations, not causal effects; people are notoriously unreliable when answering surveys; and regression in that the models are difficult to estimate and validate.
Leave a Reply